Afraid of AI? It thinks it knows that too...
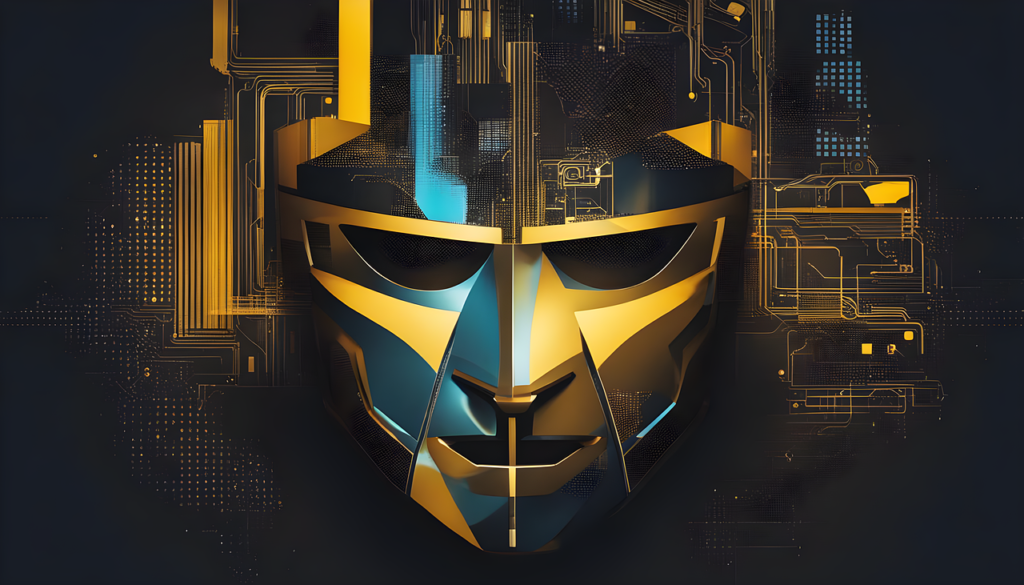
The fear of artificial intelligence (AI) is a common sentiment that has been expressed by many people. Several factors contribute to this fear, and it’s important to note that individuals may have varying reasons for feeling apprehensive about AI. Some of the common reasons include:
Job Displacement: Concerns about job displacement and automation are prevalent. The fear that AI and automation could replace human jobs, particularly in routine or repetitive tasks, has led to anxiety about employment prospects.
Lack of Control: The perceived lack of control over AI systems raises fears. If AI systems become highly autonomous and make decisions without clear human oversight, there is a concern that they could act in ways that are unpredictable or contrary to human values.
Privacy and Surveillance: The use of AI in surveillance and data analysis has raised privacy concerns. The fear that AI systems might be used to monitor individuals without their knowledge or consent contributes to apprehension.
Ethical Concerns: Ethical considerations surrounding AI, including biases in algorithms, discriminatory outcomes, and the potential for misuse, evoke fears. The idea that AI systems could perpetuate or even amplify societal biases is a significant concern.
Loss of Humanity: Some individuals fear that as AI becomes more advanced, it might lead to a loss of human connection, empathy, and the personal touch in various aspects of life, including interpersonal relationships and customer service.
Security Risks: Concerns about the security of AI systems exist. If AI systems are vulnerable to hacking or manipulation, there is a fear that they could be used for malicious purposes, leading to potential harm.
Unintended Consequences: The fear of unintended consequences arises from the complexity and unpredictability of advanced AI systems. People worry about unforeseen outcomes or scenarios that could result from the deployment of highly sophisticated AI.
Dependence on AI: A fear of over-dependence on AI for decision-making and critical tasks exists. Relying too heavily on AI without adequate human oversight may lead to situations where humans are ill-prepared to handle problems if AI systems fail.
Existential Threat: The notion of AI reaching a level of intelligence where it surpasses human capabilities and potentially poses an existential threat is a concern for some. This concept, often referred to as the “singularity,” raises questions about the control and impact of superintelligent AI.
Cultural and Psychological Factors: Cultural factors, often influenced by portrayals of AI in media and popular culture, can contribute to fear. Depictions of malevolent or uncontrollable AI in movies and literature can shape public perceptions.
It’s important to address these concerns through transparent development, ethical considerations, and responsible deployment of AI technologies. Education and open dialogue about AI’s capabilities and limitations can also help alleviate fears and foster a more informed understanding of the technology.
Current Uses of AI
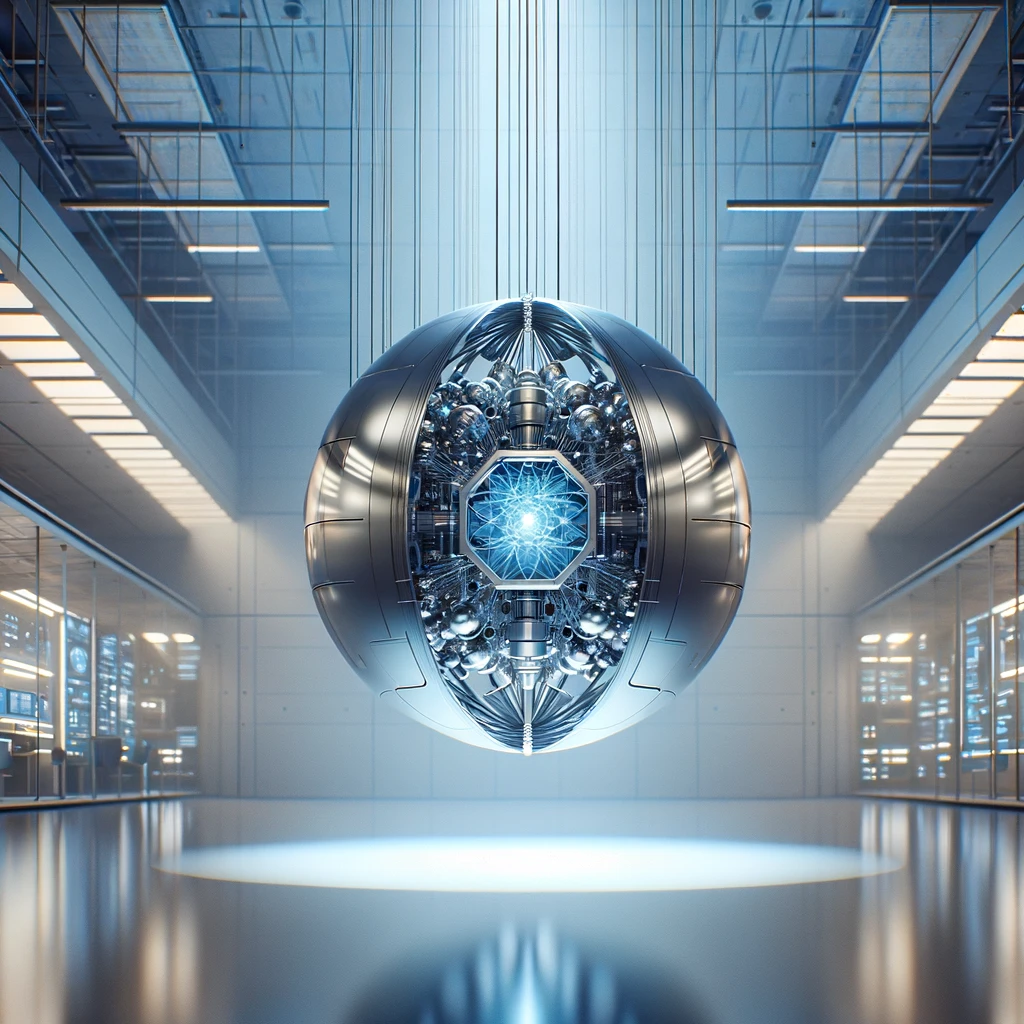
Artificial Intelligence (AI) is a broad and rapidly evolving field that encompasses a variety of technologies and applications. Here are some common capabilities and tasks that artificial intelligence can perform:
Image and Speech Recognition: AI systems can analyze and recognize patterns in images and speech. This is used in applications such as facial recognition, object detection, and speech-to-text conversion.
Natural Language Processing (NLP): NLP enables machines to understand, interpret, and generate human language. It is used in chatbots, language translation, sentiment analysis, and text summarization.
Machine Learning: AI systems can learn from data and improve their performance over time. This includes various machine learning techniques such as supervised learning, unsupervised learning, and reinforcement learning.
Decision-Making: AI systems can make decisions based on data analysis and predefined rules. This is applied in areas like recommendation systems, fraud detection, and autonomous vehicles.
Autonomous Systems: AI is used to develop autonomous systems that can operate without direct human control. Examples include self-driving cars, drones, and robotic systems.
Predictive Analytics: AI can analyze historical data to make predictions about future events or trends. This is applied in financial forecasting, demand planning, and risk management.
Robotics: AI plays a crucial role in robotics, enabling robots to perceive their environment, make decisions, and perform tasks. Industrial robots, robotic surgery, and household robots are examples.
Healthcare Applications: AI is used in medical image analysis, drug discovery, personalized medicine, and diagnostics. It can assist in diagnosing diseases, identifying patterns in medical data, and recommending treatment plans.
Virtual Assistants: AI-powered virtual assistants like Siri, Google Assistant, and Alexa use natural language processing to understand and respond to user queries. They can perform tasks, provide information, and control smart devices.
Gaming: AI is employed in gaming for creating intelligent non-player characters (NPCs), optimizing game environments, and enhancing the gaming experience through adaptive algorithms.
Cybersecurity: AI is used for threat detection, anomaly detection, and cybersecurity analytics to identify and mitigate security risks and attacks.
Education: AI applications in education include adaptive learning platforms, personalized tutoring, and automated grading systems.
Language Translation: AI-powered language translation services use machine learning to improve accuracy and enable real-time translation between languages.
Financial Services: AI is used in the financial industry for fraud detection, algorithmic trading, credit scoring, and customer service.
Climate Modeling: AI is applied to analyze large datasets in climate science, helping to predict climate patterns, understand environmental changes, and develop mitigation strategies.
The capabilities of AI continue to expand, and ongoing research and development are driving innovation in various domains. While AI has made significant advancements, it’s important to consider ethical and societal implications and ensure responsible and fair deployment of these technologies.
Training AI
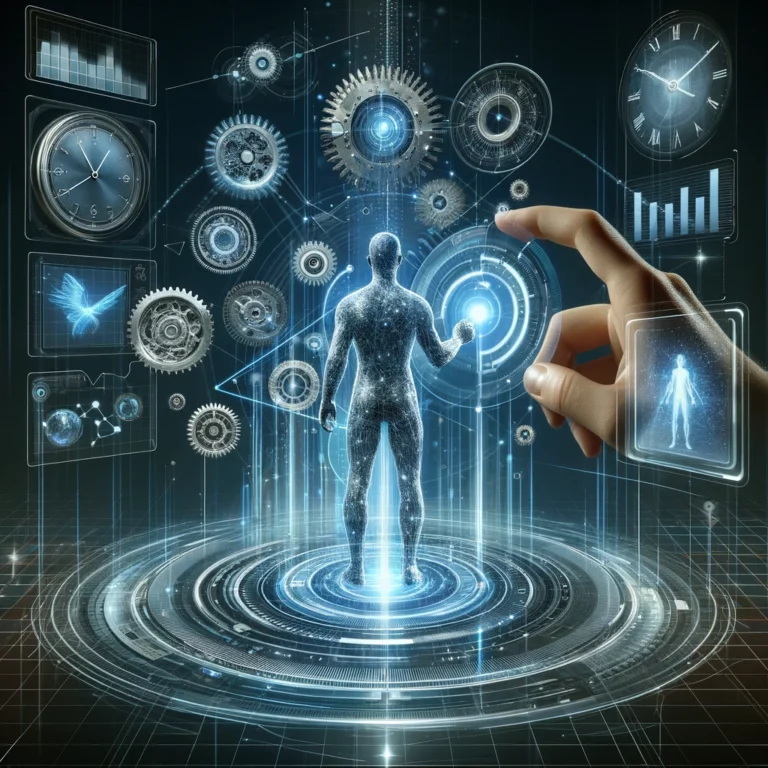
AI training involves the process of teaching artificial intelligence systems to perform tasks or make decisions by exposing them to data. There are several types of AI training, each suited to different goals and applications. Here are some common types of AI training:
Supervised Learning: In supervised learning, the AI model is trained on a labeled dataset where the input data is paired with corresponding output labels. The algorithm learns to map inputs to outputs, and the training process involves adjusting the model’s parameters to minimize the difference between predicted and actual outputs.
Unsupervised Learning: Unsupervised learning involves training AI models on datasets without labeled output. The system discovers patterns, structures, or relationships within the data without explicit guidance. Clustering and dimensionality reduction are common tasks in unsupervised learning.
Reinforcement Learning: Reinforcement learning involves training an AI agent to make decisions in an environment. The agent receives feedback in the form of rewards or penalties based on its actions. Through trial and error, the agent learns to maximize rewards and improve its decision-making.
Semi-Supervised Learning: Semi-supervised learning is a combination of supervised and unsupervised learning. It uses a dataset with both labeled and unlabeled examples, allowing the model to benefit from labeled data while also exploring the patterns in unlabeled data.
Self-Supervised Learning: Self-supervised learning involves training models using the data’s inherent structure or relationships without external labels. The model generates its own labels by creating tasks based on the input data. For example, predicting missing parts of an image or context in natural language processing.
Transfer Learning: Transfer learning involves training a model on one task and then applying the learned knowledge to a different, but related, task. This is especially useful when labeled data is limited for the target task.
Meta-Learning: Meta-learning, or learning to learn, involves training models to adapt quickly to new tasks with minimal data. The model learns a set of parameters that generalize well across various tasks.
Multi-Instance Learning: In multi-instance learning, the training data consists of bags containing multiple instances. The model learns to make predictions at the bag level rather than the instance level.
Online Learning: Online learning involves continuously updating a model as new data becomes available. This is especially useful in situations where the model needs to adapt to changing conditions.
These types of AI training cater to different scenarios and application domains. The choice of training approach depends on the nature of the task, the availability of labeled data, and the desired outcomes.
AI at Your Service
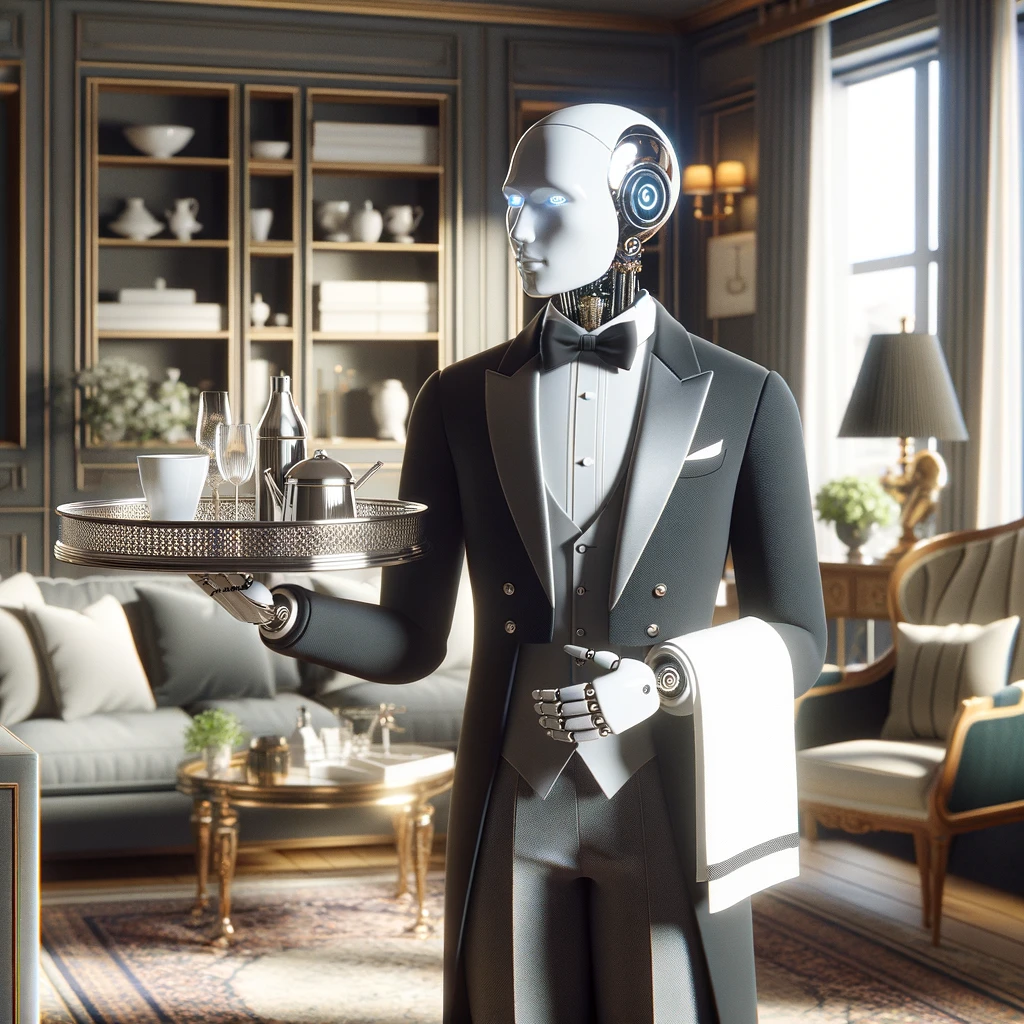
Artificial Intelligence is a powerful tool causing immense change at lightning speed over the world. If you are concerned about where this tidal wave of technology is going, take positive action now as the development of this technology is only going to continue picking up speed. AI is already so prominent in our daily lives and is being implemented across many areas of technology, whether we are using it directly ourselves or are the unwitting subjects of AI being used on us. Rather than succumbing to temptations to feel threatened by media’s portrayals of evil AI trying to take over the world, consider how learning about this evolving technology can aid you in advancing yourself and your abilities and even your business. So, rather than feeling like AI is going to take your job from you, how can you use AI to advance in your job or add to your business model?
Toughen Up
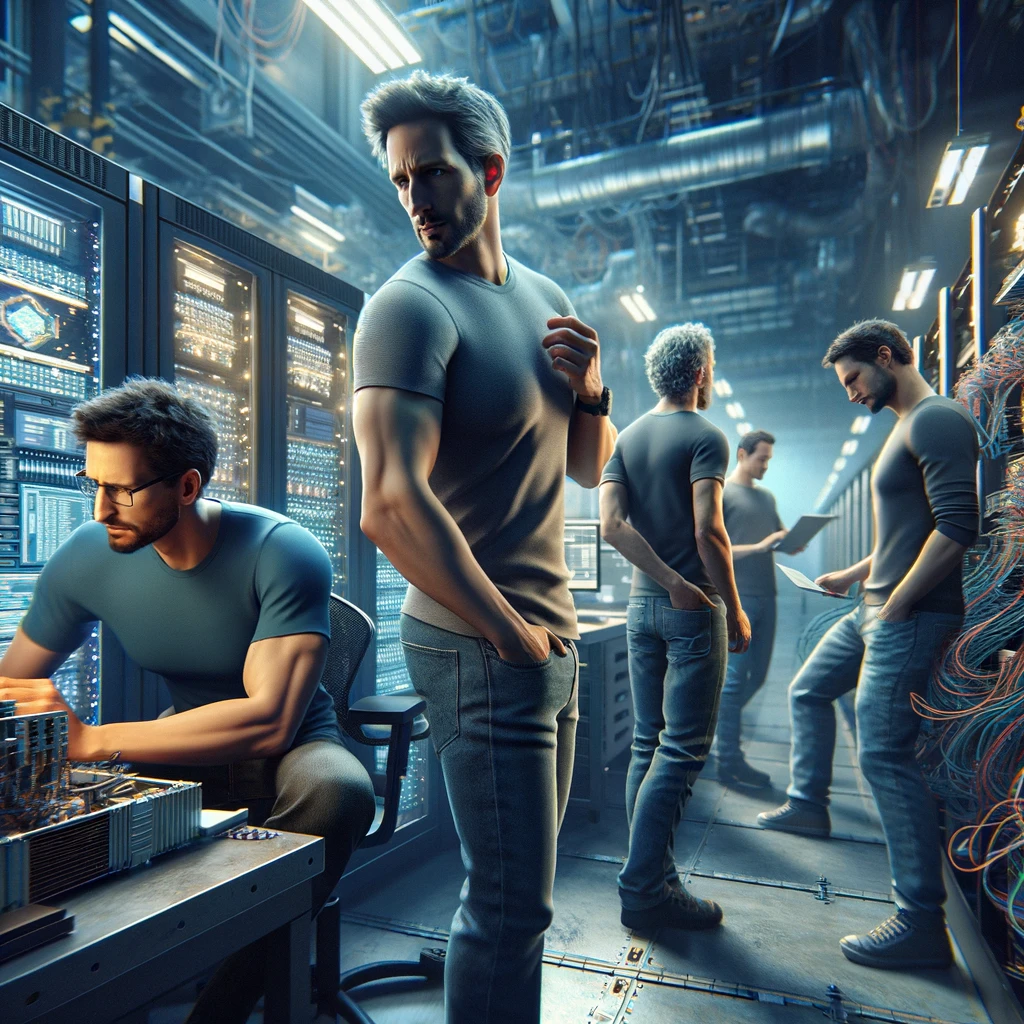
The perception that one needs to be emotionally resilient or tough to work with artificial intelligence (AI) may stem from several factors:
Complexity of AI Technologies: AI technologies can be complex and require a deep understanding of technical concepts, algorithms, and methodologies. Dealing with such complexity may require resilience to navigate challenges, setbacks, and uncertainties inherent in working with cutting-edge technology.
High-Stakes Environments: In certain industries or applications, the deployment of AI systems can have significant implications, such as in healthcare, finance, or autonomous vehicles. Working with AI in high-stakes environments may require individuals to handle pressure, make critical decisions, and cope with the potential consequences of AI-related outcomes.
Rapid Technological Change: The field of AI is characterized by rapid technological advancements and continuous innovation. Keeping pace with these changes and adapting to new tools, techniques, and methodologies may require individuals to be adaptable, flexible, and resilient in the face of uncertainty and ambiguity.
Ethical and Societal Implications: AI technologies raise complex ethical and societal questions related to privacy, bias, job displacement, and the potential impact on human well-being. Addressing these issues requires individuals to grapple with ethical dilemmas, engage in nuanced discussions, and navigate conflicting viewpoints with emotional intelligence and resilience.
Challenges of Implementation: Implementing AI solutions within organizations or integrating them into existing systems can pose various challenges, including resistance to change, technical obstacles, and cultural barriers. Overcoming these challenges may require individuals to demonstrate resilience, perseverance, and leadership skills to drive successful AI initiatives.
Handling Failures and Setbacks: Like any technology, AI systems are not immune to failures, errors, and unexpected outcomes. Dealing with these failures in a constructive manner, learning from mistakes, and iterating on solutions require emotional resilience and a growth mindset to bounce back and continue making progress.
Overall, while working with AI can be intellectually stimulating and rewarding, it also presents unique challenges that may require individuals to cultivate emotional resilience, adaptability, and perseverance to succeed in this dynamic and rapidly evolving field.
Character Building: Emotional Resilience
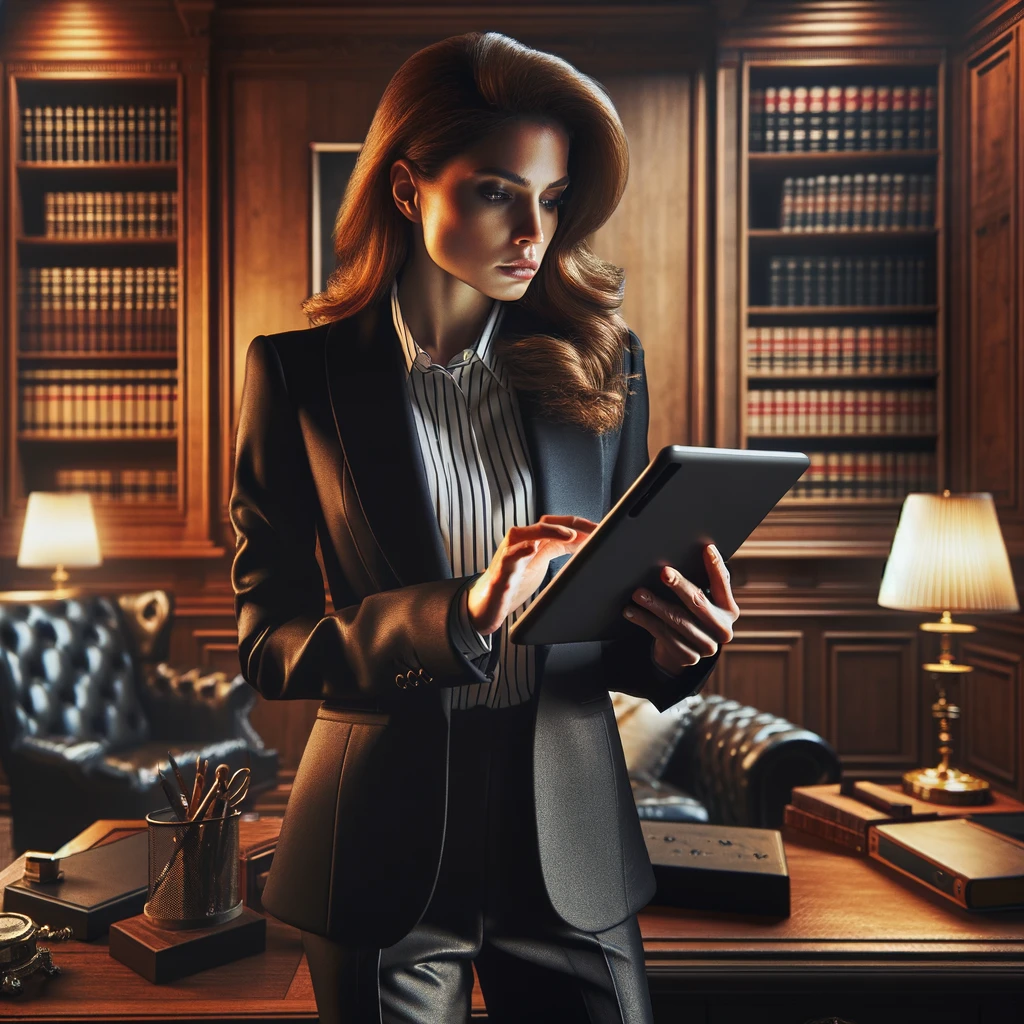
The interaction between typical users and AI systems can lead to situations where emotional resilience becomes important. Here’s how:
Misunderstandings and Misinterpretations: AI systems, particularly those based on natural language processing (NLP) or machine learning, may sometimes misinterpret user inputs or fail to understand context accurately. This can lead to frustration or confusion on the part of users, especially if they receive unexpected or irrelevant responses from the AI system. Developing emotional resilience can help users manage their reactions to these misunderstandings and maintain a positive attitude towards using AI despite occasional hiccups.
Difficult Feedback: When interacting with AI systems for tasks like learning or receiving recommendations, users may receive feedback that is critical or challenging to accept. For example, an AI-powered educational tool might provide feedback on areas where the user needs improvement, or a recommendation system might suggest content that contradicts the user’s preferences. Building emotional resilience can help users process and internalize this feedback constructively, using it as an opportunity for growth rather than feeling discouraged or demotivated.
Impact of AI on Daily Life: As AI technologies become increasingly integrated into daily life, people may encounter situations where AI decisions or recommendations have tangible consequences. For instance, AI-powered hiring systems may influence job opportunities, or AI-driven financial algorithms may impact investment decisions. In such cases, emotional resilience can help individuals cope with the potential stress or uncertainty associated with relying on AI for important decisions, while also advocating for transparency and accountability in AI systems.
Overall, while AI has the potential to enhance efficiency, convenience, and productivity in various domains, its integration into everyday life can also pose challenges that require individuals to develop emotional resilience. By cultivating skills such as patience, adaptability, and self-awareness, users can navigate interactions with AI systems more effectively and harness their benefits while mitigating potential drawbacks.
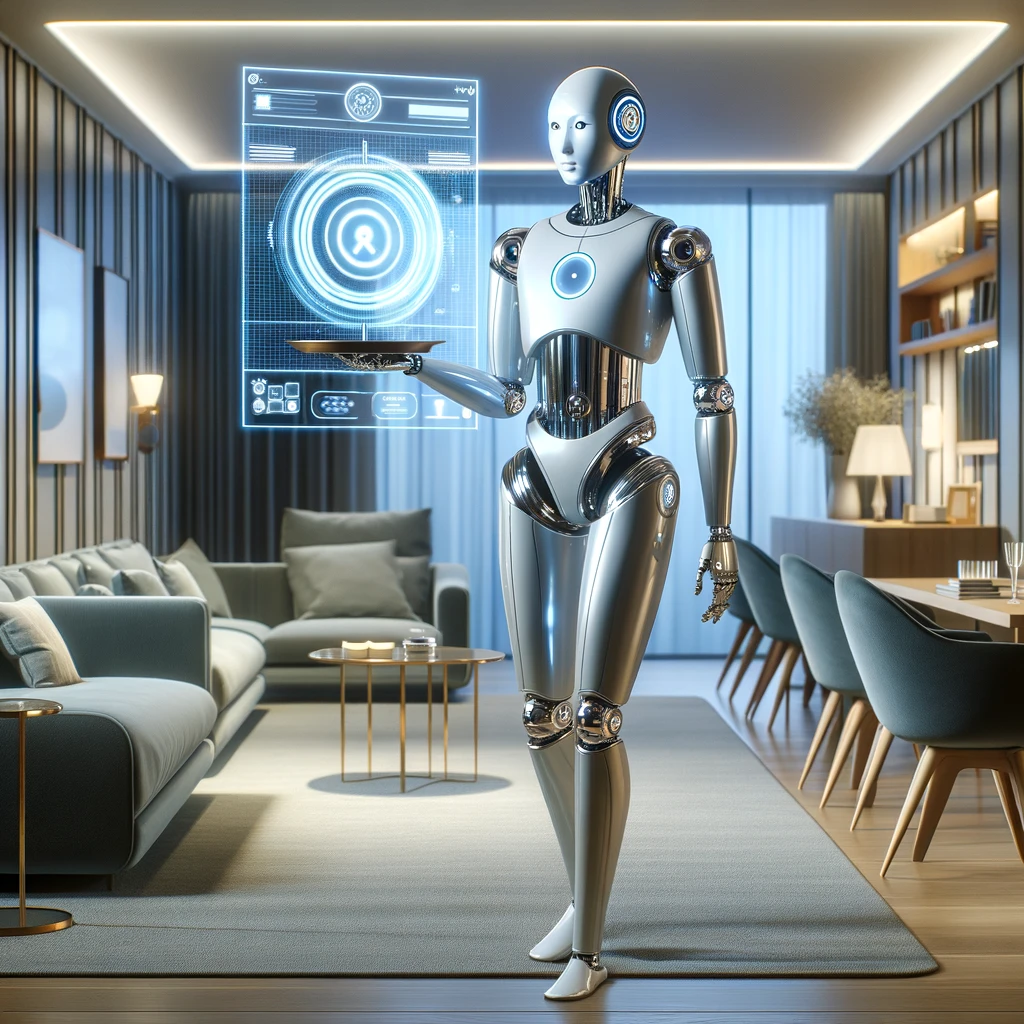